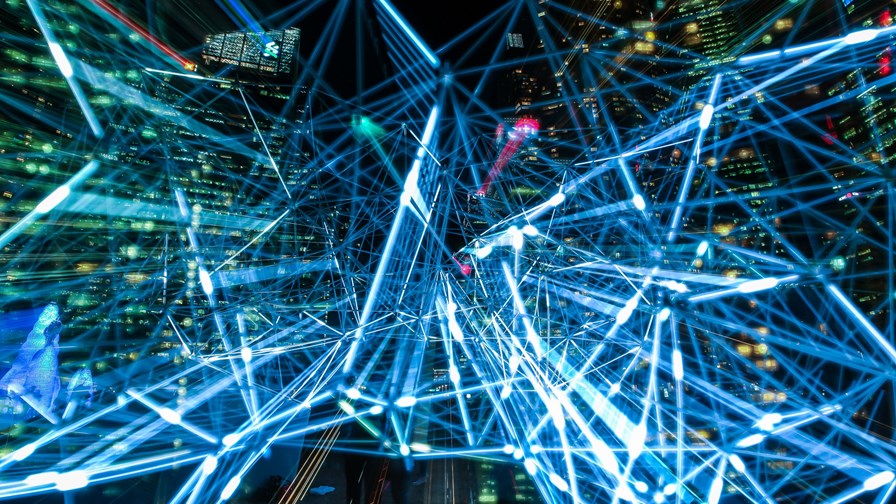
- Data Pipeline Best Practices
- Big data, big mistake
- You have to do it to get it
There is a lot of buzz in telecoms about using analytics and artificial intelligence (AI), and especially machine learning, to enable much greater operational and network agility and automation as we move into the era of 5G. Yet all the AI in the world is useless without timely access to clean, actionable data across the organisation, which remains a challenging issue almost everywhere, even though we’ve been talking about big data since 2005.
Communications Service Providers (CoSPs) have more data than most other businesses. For many years they viewed data as a by-product or tool for a specific purpose rather than an asset in its own right that could transform their businesses. Building a data-driven business is what digital transformation is all about. It is key to enabling CoSPs to become digital service providers (DSPs), which means not only gaining far greater insight into their network operations, IT, business models and customers than ever before, but using that information to change them radically.
Being data-driven makes network operations and IT more agile, adaptable and streamlined. In turn this means they can support new business models (including monetising the data itself) and become more customer centric than was ever possible before – in line with what customers have come to expect from all service providers from their online experiences.
In line with Moore’s Law, (find out how it’s still applicable and evolving 50 years on) was the cost of computing and storage kept falling sharply, and CoSPs were generating unprecedented amounts of data. They were keen to extract value from it using analytics and the notion of the data life cycle was born, as shown below.
This didn’t work well for most of them for various reasons, including the big technological and organisational challenges involved in progressing from Acquisition to Use. We will look at those issues in more detail in this series of four articles, but the most fundamental problem was that they typically started with what they saw as the beginning of the life cycle – data acquisition.
Big data, big mistake
Eventually it became clear that it is impossible to find something if you don’t know what you’re looking for or why you’re searching for it. Organisations first need to figure out what they want to achieve, then work ‘backwards’ to discover the best way of gaining the desired outcome. CoSPs need to figure out which data they need, for what purpose or purposes, and how fast they need it if it is to be truly useful. In short, the data life cycle is holistic – what happens in each stage has a massive influence on what is possible in the next.
CoSPs often talk the challenges of data in terms of the five ‘Vs’, meaning volume, variety, velocity, veracity and value. It says it all that for many years, we talked about four Vs of big data, missing off ‘value’ because we were focused on the processes, not the outcome or business case.
Volume | Variety | Veracity | Velocity | Value |
CoSPs generate immense amounts of data from sources including their networks, services and customers. | The data comes in many formats, is often incomplete, siloed and incompatible. | Data should be a ‘single source of truth’ – the cleansing processes needed to get it to that point are far from simple. | This is no longer about how fast data is produced – that’s a given – but how fast you can act on it in real time or close to it, if necessary. | Value is often derived from mixing data sets, that were designed to be discrete, to improve ops, profit, CX or TTM. |
Telefónica Group, however, grasped the potential value of data early, back in 2012, and decided the best way of extracting value from it was by establishing a central hub, LUCA, to coordinate data activities within its different businesses. The company identified key use cases, which were then deployed and monitored both from a business perspective and the physical, network point of view, and the lessons learned applied elsewhere.
Increasingly, the Group’s operations and business revolve around four platforms:
- Physical assets, such as the network and shops
- The operating system and business system software (OSS/BSS) and the whole IT stack
- Products and services, such as TV, IoT, financial services, and internal operations
- AI and big data
It has been successful – in October 2018, Forrester wrote a report, LUCA, Telefónica Data Unit, is positioned as a Leader noting “the strength of Telefónica's offering lies in data from its 350 million subscribers across 17 countries, including call data records, detailed data records, social network analytics, location, and subscriber CRM data.” Nevertheless, as this article from Telefónicawatch explains, it is under huge pressure from banks and analysts to speed up the delivery of benefits from its digital transformation.
At the FutureNet World conference in London in March, Richard Benjamins, Data and AI Ambassador, Telefónica, gave a presentation, How network automation and artificial intelligence (AI) are enabling new digital business models and services using data. This offered valuable insight into why, despite progress, deploying AI is far from quick or easy.
He gave the example of having set up use cases for data analytics in one country, the company moved to replicate them in others. Benjamins said, “Instead of going from, let's say, six months to two months, we went from six months to five and a half months, and then five months.” This was because the data was different and accessed differently in each operation”. Lack of scalability is one of the biggest stumbling blocks in deploying AI.
You have to do it to get it
Intel’s approach has considerable similarities to that of Telefónica. In its quest to transform itself into a digital corporation, it created a framework to identify, prioritise, execute, and continuously monitor and review data maturity. To this end, it established a Corporate Data Office (CDO) early in 2017 as a “one-stop data shop” to consolidate the work of cultivating a data-first mindset for each of Intel’s businesses.
The CDO was initially established to improve the management of Intel’s enormous data assets, but its role was expanded because all the top trends in technology – from autonomous vehicles to blockchain, edge computing, natural language processing and virtual reality – rely on analytics and AI. As our world continues to generate unprecedented amounts of data, there are opportunities to make systems more efficient and create entirely new, immersive services.
The challenge remains how to translate raw data into useful insights, hence Intel wanted to embark on that journey for its own sake, but also to gain first-hand knowledge of how to bring about digital transformation so it would well placed to help its customers with theirs.
As we’ve summarised in this article, much of the answer lies with an intelligently managed data lifecycle, built on the solid foundation of understanding what the business goals your data-first strategy is designed to achieve.
This is the first in a series of four articles; the next one looks at successfully ingesting data from multiple sources and is entitled "Moving more to the edge doesn’t mean bigger risks".
Email Newsletters
Sign up to receive TelecomTV's top news and videos, plus exclusive subscriber-only content direct to your inbox.