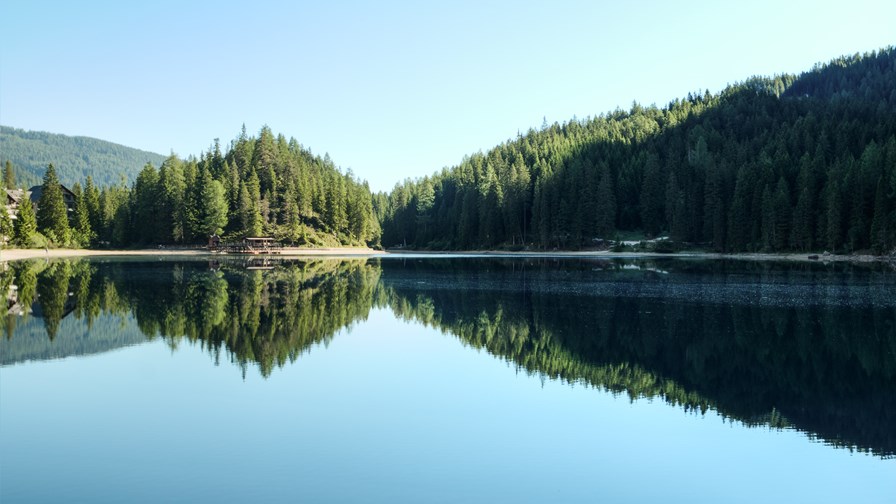
- The data estate
- A big lesson
- Securing success
- Best practices and benchmarking
- What’s next?
Communications service providers (CoSPs) are striving to streamline their internal processes and work out how best they can apply analytics, automation, machine learning and other kinds of artificial intelligence (AI) to their massive amounts of data.
They want to release value by combining data sets and by making their operations and businesses data driven, and are only too aware that the boom in connected devices will exponentially increase the amount of data they produce every single day.
This is why they are looking for a more standardised strategy for data management. As they have so much unstructured data, data lakes are particularly attractive: the lakes have flat architectures and use Hadoop or object-based storage to store many different kinds of raw data, and are extensible and scalable.
However, using a data lake as a single repository doesn’t automatically enable CoSPs to extract value from their often incomplete and incompatible data from many sources. The first hurdle is pulling all the data that is needed into the lake, but before this process begins, CoSPs need to think about how they store data in the lake in a way that makes it accessible and useable, or they risk creating a data swamp: the aim is to create a single source of truth from the data, not muddy the waters.
CoSPs need to establish processes for auditing the data and create accurate metadata before the data is stored. Knowing what data is stored where is important for operational efficiency, but also to comply with regulation. For example, part of the European Union’s General Data Privacy Regulation (GDPR) is the right to be forgotten, which makes it essential to know where all the relevant data is located.
To ensure data quality, companies must catalogue the data and create business rules to validate, match and cleanse the data. Third-party databases can help find and complete customers’ data, and increasingly AI and automation will play an important role in data cleansing so that as the lake is populated, more and more of it can be cleansed without human intervention.
It is important to ensure that quality enforcement practices are put in place because if they are not, CoSPs will experience serious obstacles further down the line. They need to deploy solutions for data query and transformation within the data lake, such as Hive, the data warehouse software project built on top of Hadoop for providing data query and analysis. Hive can query data stored in various databases and file systems that integrate with Hadoop.
Each part of creating, maintaining and operationalising a data lake is complex and difficult, but the payback for getting it right is huge and foundational to the success of any company that is striving to become data-driven as Intel’s own IT has proved.
The data estate
Intel IT operates 56 data centres with a total capacity of 86 megawatts, housing about 289,000 servers to provide computing resources for more than 106,000 employees. To support critical business functions – design, office, manufacturing and enterprise (DOME) – and operate the data centres as efficiently as possible, Intel IT embarked on a multi-year evolution strategy.
The strategy enabled the company to improve data management to boost growth and reduce unit cost without increasing budget: between 2010 and 2018 it generated savings of more than $2.8 billion. Intel IT views and runs all the data centres and their underlying infrastructure like factories, with a disciplined approach to change management.
A big lesson
Intel IT came to see that a one-size-fits-all architecture is not the best approach for its business functions, having worked closely with business leaders to understand their requirements. The company decided to invest in vertically integrated architecture solutions to meet the specific needs of individual business functions.
For example, Design engineers run more than 166 million compute-intensive batch jobs every week, which can take minutes or days, depending on their parameters. As design engineers need access to data frequently and quickly, Intel instead invested in clustered and higher performance storage, that is attached to the network, combined with caching on local storage for our high-performance computing needs. Also, it uses storage-area networks for particular storage needs like databases.
In contrast, Intel’s Office and Enterprise environments rely primarily on a storage-area network, with limited network-attached storage for file-based data sharing.
Manufacturing is a different beast: IT systems must be available 24/7 so Intel deployed dedicated data centres that are co-located with the plants.
Securing success
Whatever their operational differences, every part of the business needs to be secured, as part of the initial design and deployment. Encryption plays an important part in securing data, both in flight and at rest, but if deployed poorly it can have an adverse effect on performance, slowing everything down.
As Intel continues with the transformation of its own data centre infrastructure, it deploys Advanced Encryption Standard-New Instruction (AES-NI) and other hardware-enabled technologies on the Intel Xeon family of processors to secure data while minimising the impact on performance, and of course the technology gets better all the time.
Best practices and benchmarking
In fact, a critical element of Intel IT’s strategy, and one that is behind much of its success, is that every year it applies breakthrough improvements in technologies, solutions and processes across its data centre estate and uses three key performance indicators (KPIs) to define a model of record (MOR) for the year. A good example of a breakthrough technology is the Intel Optane DC persistent memory, which sits between the DRAM on servers and solid-state drives to provide near-DRAM performance at less cost than DRAM.
Optane DC Persistent Memory doesn’t replace DRAM, but it supplements it, allowing enterprises, CoSPs and cloud service providers to bring larger amounts of data into memory and closer to the central processing unit, which, in turn, gives users with much faster access to data.
Another example is Intel’s disaggregated server design allows independent refresh of CPU and memory without replacing other server components. This results in faster data centre innovation and at least 44% cost savings compared to a full-acquisition refresh.
The KPIs to monitor and measure performance of data management for the MOR are:
- best achievable quality of service (QoS) and service-level agreements (SLAs);
- the lowest achievable unit cost; and
- the highest achievable use of resources
The company sets investment priorities based on those KPIs to move toward the MOR goals. An example of a priority arising from the KPIs and MOR is tiered storage – a super-efficient way to match storage resources to the data workload as data volumes increase. For Design storages, Intel has implemented a four-tier approach to improve utilisation levels, better meet SLAs, and reduce the total cost of ownership. The tiers for Design storage servers are based on performance, capacity, and cost.
What’s next?
Like its CoSP customers, Intel is always working to close the gap between current achievements and the best possible scenario. Over the next three years, to 2022, it will continue to transform its data centre infrastructure with the goal of providing unprecedented quality-of-service levels while reducing the total cost of ownership for business applications, making IT operations ever more efficient and being environmentally responsible.
As we stressed in the first article in this series – The end is where to begin with the data life cycle – whatever KPIs and tactics are put in place as part of the strategy, they are only tools to help achieve the business goal. And at this stage of preparing and processing the data in particular, CoSPs must resist getting bogged down in how they are transforming data management and losing sight of the why.
This is the third article in a series of four looking at the data lifecycle. You can read about the start of the cycle here and the next in the series, on extracting value from data here.
Email Newsletters
Sign up to receive TelecomTV's top news and videos, plus exclusive subscriber-only content direct to your inbox.